CHI.2021
Repeated exposure to poor air quality in indoor environments such as office, home, and classroom can have substantial adverse effects on our health and productivity. The problem is especially recognized in closed indoor spaces shared by several people.On the other hand, sustainability concerns restrain the acceptable architectural methods for supplying fresh air in buildings.
We have studied the evolution of carbon dioxide level in office-meeting spaces, during 1007 meeting sessions. The collected data is employed to examine machine learning models aimed to indicate the CO2 evolution pattern and to forecast when fresh air should be supplied. In addition, to gain insight into the relations and interdependencies of social factors in meetings that may influence the users' perception of an interactive solution, we have conducted a series of online surveys. Building on the results of the two studies, a design solution is proposed that predicts the evolution of air quality in naturally-ventilated meeting rooms and engages the users in preventive actions when risk is forecast.
The Complexity of Indoor Air Quality Forecasting and the Simplicity of Interacting with It – A Case Study of 1007 Office Meetings
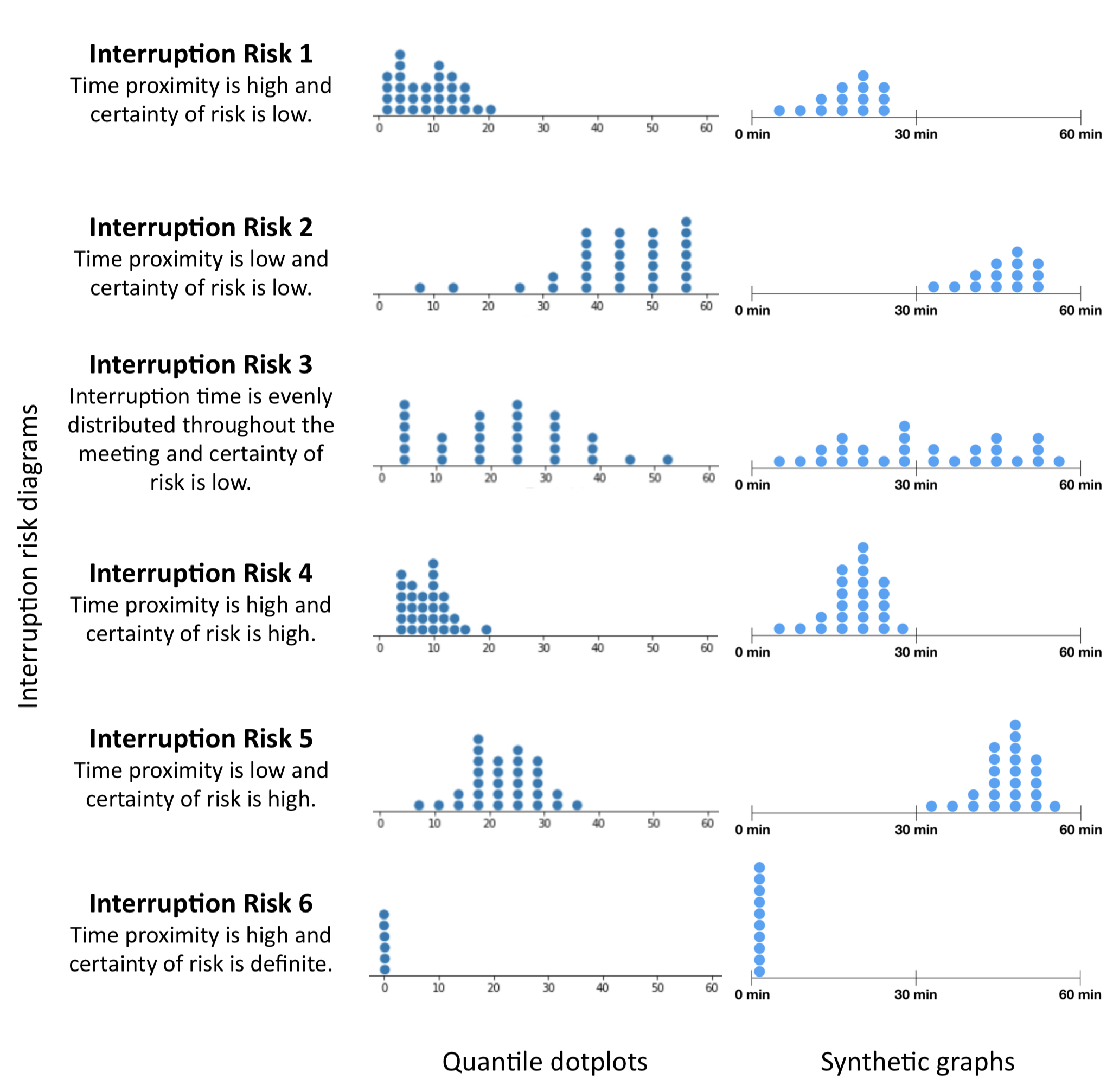

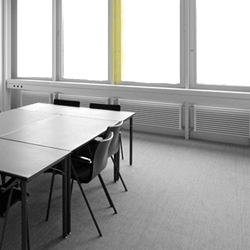
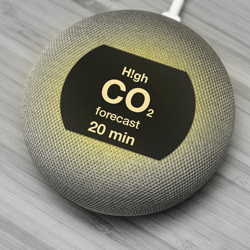